Main Conclusion
Increasing the number of contacts with clients during the campaign does not necessarily result in a higher probability of positive contributions to the "deposit." Therefore, as a strategy to enhance marketing effectiveness, it is considered desirable to minimize contact with customers while increasing the contact duration. Particularly, marketing outcomes do not seem to be significantly influenced by the customer's age. It appears more favorable to focus on customers who were successful in previous marketing campaigns and students.
Summary for Results by Analysis Procedures
Step01. Data Extraction
- Missing Value & Duplication Inspection: It has been confirmed that there are no data missing or duplicates, and the the training dataset consists of 45,211 instances while the test dataset 4,521 instances.
Step02. Exploratory Data Analysis
- Descriptive Statistics: The datasets consists of a total of 17 columns, including 10 columns of string type and 6 columns of integer or floating-point type.
- Exploration of independence between features: It has been confirmed that there are no explainatory variables (16 columns) that maintain independence.
- Exploration of association with target feature: Based on decision tree and association analysis, it is inferred that the attributes "duration", "balance", "poutcome", and "month" are the major factors that determine the "deposit"(target feature).
Step03. Linear Relationship Analysis
- Regression Analysis: It has been observed that the attributes "default", "marital", "load", "education", and "age" exhibit a strong multicollinearity. As a result, the attribute that contributes most positively to the "deposit" is "duration". Specifically, when the job is "student" or the previous marketing campaign was successful, there is a higher probability of positive contribution to the "deposit." On the other hand, the attribute "campaign" has a negative contribution. When the job is "student" and the education level is "tertiary," the probability of positive contribution to the "deposit" is low.
- Covariance Analysis: It has been observed that the combinations of explanatory variables with positive correlation are ("job", "education"), ("pdays", "previous"), ("pdays", "poutcome"), and ("previous", "poutcome"). On the other hand, the combination of ("age", "marital") has been identified to have a negative correlation. Futhermore, it has been determined that the target variable has a positive correlation with "duration", "poutcome", and "month".
- Exploratory Factor Analysis: It has been observed that "pdays", "previous", and "poutcome" share a common factor related to previous campaigns. Additionally, it has been confimed that "age" and "marital" also share customer demographic attributes. Moreover, "age", "job", "education", "housing", "contact", and "month" have been found to interact synergistically with the "deposit".
Step04. Predictive Modeling and Evaluation
- Effect validation by data preprocessing scenarioes: Based on the recall score and the LDA model, four main preprocessing approaches were explored. It appears that the prior effect, upsampling, addressing modeling assumptions approaches have shown positive effects. However, outliers handling seems to have demonstrated no significant impact on the results.
- Validation baseline for training models: After conducting two rounds of testing on the sampled data, it has been observed that the baseline model yielded low recall scores. In order to improve the recall score, various modeling approaches are being explored. LDA, KNN, and GBC models are considered effective for enhancing performace.
- AI Model Evaluation: Great job on improving the recall score of the KNN baseline model from 0.3647 to 0.9674. However, it's important to note that there is a trade-off effect as the precision decreased from 0.5460 to 0.4448.
Step05. Summary for Results by Analysis Procedures
Step06. Main Conclusion
Predictive Modeling and Evaluation
AI Model Evaluation
Final test result
model | total | TP | TN | FP | FN | accuracy | precision | recall | f1 |
logisticregression | 4521 | 374 | 3500 | 500 | 147 | 0.856890 | 0.427918 | 0.717850 | 0.536201 |
lineardiscriminantanalysis | 4521 | 359 | 3571 | 429 | 162 | 0.869277 | 0.455584 | 0.689060 | 0.548510 |
svc | 4521 | 361 | 3200 | 800 | 160 | 0.787658 | 0.310939 | 0.692898 | 0.429251 |
kneighborsclassifier | 4521 | 504 | 3371 | 629 | 17 | 0.857111 | 0.444837 | 0.967370 | 0.609432 |
extratreeclassifier | 4521 | 492 | 1959 | 2041 | 29 | 0.542137 | 0.194236 | 0.944338 | 0.322200 |
gradientboostingclassifier | 4521 | 520 | 637 | 3363 | 1 | 0.255917 | 0.133917 | 0.998081 | 0.236149 |
Validation baseline for training models
Modeling
probabilistic generative models: GNB, LDA, QDA, ...
main objective: probabilistic interpretation for conditional distribution of features on data
- selected validation models: 'linear discriminative analysis' model
- checking point: conditional independence viloation of the distribution of individual classes
probabilistic discriminative models: KNN, DT, RF, Ensembles, Logit, SVM, NN, ...
main objective: probabilistic interpretation for information quantity(i.e. information gain) of each features on data
- selected validation models: 'k-nearst neighbors', 'extra tree', 'gradient boosting ensemble'
- checking point: the hard or soft decision boundary between classes
- selected validation models: 'logistic regression', 'support vector machine' model
- checking point: feature independence viloation
Hyper-parameter ranges to prevent overfitting during learning
Model | hyper-parameter1 | hyper-parameter2 | hyper-parameter3 |
Logistic | C: [0.001, 0.005, 0.007] | ||
LDA | priors: [(0.1, 0.9), (0.2, 0.8), (0.3, 0.7)] | ||
SVC | C: [0.01, 0.05, 0.07] | ||
KNN | n_neighbors: [20, 30] | leaf_size: [30, 50, 100] | |
ETC | min_impurity_decrease: [0.01, 0.05, 0.1] | max_depth: [10, 20, 30] | |
GBC | min_impurity_decrease: [0.01, 0.05, 0.1] | n_estimators: [10, 30, 50] | subsample: [0.7, 0.8, 1] |
Transformers for data preprocessing
Objective : preprocessing |
Normality and decision boundry secure |
Numericalization | Feature selection | Dimensionality reduction |
Feature diversification |
Model | Powertransformer | OnehotEncoder | SelectPercentile | PCA | SplineTransformer |
Logistic | O | X | O | X | O |
LDA | O | X | O | X | O |
SVC | O | O | O | X | O |
KNN | O | O | O | X | O |
ETC | O | O | O | X | O |
GBC | O | O | O | X | O |
Validation Result
2nd test result
preprocessing for stratified sampling (frac=.1 & 3 repeated * 5 fold)
- upsampling SMOTE
- custom preprocessing for continous feature
model | total | TP | TN | FP | FN | accuracy | precision | recall | f1 |
logistic | 4521 | 390 | 3469 | 531 | 131 | 0.8535 | 0.4234 | 0.7485 | 0.5409 |
LDA | 4521 | 354 | 3551 | 449 | 167 | 0.8637 | 0.4408 | 0.6794 | 0.5347 |
SVC | 4521 | 350 | 3241 | 759 | 171 | 0.7942 | 0.3156 | 0.6717 | 0.4294 |
KNN | 4521 | 415 | 3041 | 959 | 106 | 0.7644 | 0.3020 | 0.7965 | 0.4379 |
ETC | 4521 | 327 | 2507 | 1493 | 194 | 0.6268 | 0.1796 | 0.6276 | 0.2793 |
GBC | 4521 | 520 | 677 | 3323 | 1 | 0.2647 | 0.1353 | 0.9980 | 0.2383 |
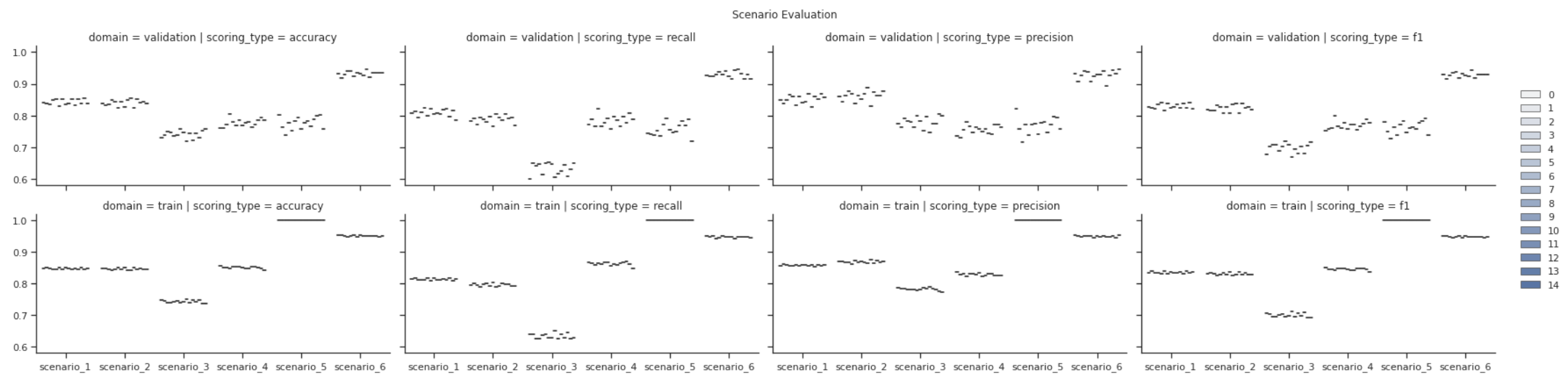
1st test result
baseline for stratified sampling (frac=.1 & 3 repeated * 5 fold)
model | total | TP | TN | FP | FN | accuracy | precision | recall | f1 |
Logistic | 4521 | 142 | 3922 | 78 | 379 | 0.8989 | 0.6455 | 0.2726 | 0.3833 |
LDA | 4521 | 198 | 3879 | 121 | 323 | 0.9018 | 0.6207 | 0.3800 | 0.4714 |
SVC | 4521 | 0 | 4000 | 0 | 521 | 0.8848 | 0.0000 | 0.0000 | 0.0000 |
KNN | 4521 | 190 | 3842 | 158 | 331 | 0.8918 | 0.5460 | 0.3647 | 0.4374 |
ETC | 4521 | 98 | 3953 | 47 | 423 | 0.8960 | 0.6759 | 0.1881 | 0.2943 |
GBC | 4521 | 221 | 3865 | 135 | 300 | 0.9038 | 0.6208 | 0.4242 | 0.5040 |
Effect validation by data preprocessing scenarioes
First, prior effect, this involves addressing the influence or prior information or biases in the data. Second, upsampling for class imbalance, to tackle class imbalance, upsampling techniques were applied to increase the representation of the minority class. Third, effects of addressing modeling assumptions, this includes addressing assumptions such as normality, standardization, and normalization to meet the modeling requirements. Lastly, outlier handling, the effect of outlier handling was examined, which involves identifying and dealing with data points that deviate significantly from the overall pattern. These four preprocessing approaches were evaluated in terms of their impact on the performance, specifically with regard to the recall score and the LDA model.
Cost-sensitive priors
Priors: effective
test_recall | |
---|---|
param_priors | |
(0.1, 0.9) | 0.9210 |
(0.2, 0.8) | 0.8801 |
(0.3, 0.7) | 0.8427 |
(0.4, 0.6) | 0.7973 |
(0.5, 0.5) | 0.7512 |
(0.6, 0.4) | 0.6948 |
(0.7, 0.3) | 0.6347 |
(0.8, 0.2) | 0.5752 |
(0.9, 0.1) | 0.4925 |
Source | SS | DF | MS | F | p-unc | np2 | |
---|---|---|---|---|---|---|---|
0 | param_priors | 0.8366 | 8 | 0.1046 | 284.0343 | 0.0 | 0.9844 |
1 | Within | 0.0133 | 36 | 0.0004 | NaN | NaN | NaN |
A(no, yes) | B(no, yes) | mean(A) | mean(B) | diff | se | T | p-tukey | hedges | |
---|---|---|---|---|---|---|---|---|---|
0 | (0.1, 0.9) | (0.2, 0.8) | 0.9210 | 0.8801 | 0.0408 | 0.0121 | 3.3652 | 0.0424 | 4.8871 |
1 | (0.1, 0.9) | (0.3, 0.7) | 0.9210 | 0.8427 | 0.0783 | 0.0121 | 6.4498 | 0.0000 | 4.9797 |
2 | (0.1, 0.9) | (0.4, 0.6) | 0.9210 | 0.7973 | 0.1237 | 0.0121 | 10.1891 | 0.0000 | 6.1375 |
3 | (0.1, 0.9) | (0.5, 0.5) | 0.9210 | 0.7512 | 0.1698 | 0.0121 | 13.9904 | 0.0000 | 8.8321 |
4 | (0.1, 0.9) | (0.6, 0.4) | 0.9210 | 0.6948 | 0.2261 | 0.0121 | 18.6331 | 0.0000 | 13.1020 |
5 | (0.1, 0.9) | (0.7, 0.3) | 0.9210 | 0.6347 | 0.2863 | 0.0121 | 23.5874 | 0.0000 | 15.2181 |
6 | (0.1, 0.9) | (0.8, 0.2) | 0.9210 | 0.5752 | 0.3458 | 0.0121 | 28.4951 | 0.0000 | 23.8565 |
7 | (0.1, 0.9) | (0.9, 0.1) | 0.9210 | 0.4925 | 0.4284 | 0.0121 | 35.3035 | 0.0000 | 26.6182 |
8 | (0.2, 0.8) | (0.3, 0.7) | 0.8801 | 0.8427 | 0.0374 | 0.0121 | 3.0846 | 0.0819 | 2.3003 |
9 | (0.2, 0.8) | (0.4, 0.6) | 0.8801 | 0.7973 | 0.0828 | 0.0121 | 6.8238 | 0.0000 | 4.0234 |
10 | (0.2, 0.8) | (0.5, 0.5) | 0.8801 | 0.7512 | 0.1289 | 0.0121 | 10.6252 | 0.0000 | 6.5521 |
11 | (0.2, 0.8) | (0.6, 0.4) | 0.8801 | 0.6948 | 0.1853 | 0.0121 | 15.2679 | 0.0000 | 10.4294 |
12 | (0.2, 0.8) | (0.7, 0.3) | 0.8801 | 0.6347 | 0.2454 | 0.0121 | 20.2222 | 0.0000 | 12.7315 |
13 | (0.2, 0.8) | (0.8, 0.2) | 0.8801 | 0.5752 | 0.3050 | 0.0121 | 25.1298 | 0.0000 | 20.2030 |
14 | (0.2, 0.8) | (0.9, 0.1) | 0.8801 | 0.4925 | 0.3876 | 0.0121 | 31.9383 | 0.0000 | 23.2960 |
15 | (0.3, 0.7) | (0.4, 0.6) | 0.8427 | 0.7973 | 0.0454 | 0.0121 | 3.7392 | 0.0164 | 1.8512 |
16 | (0.3, 0.7) | (0.5, 0.5) | 0.8427 | 0.7512 | 0.0915 | 0.0121 | 7.5406 | 0.0000 | 3.8515 |
17 | (0.3, 0.7) | (0.6, 0.4) | 0.8427 | 0.6948 | 0.1479 | 0.0121 | 12.1833 | 0.0000 | 6.6599 |
18 | (0.3, 0.7) | (0.7, 0.3) | 0.8427 | 0.6347 | 0.2080 | 0.0121 | 17.1376 | 0.0000 | 8.8779 |
19 | (0.3, 0.7) | (0.8, 0.2) | 0.8427 | 0.5752 | 0.2675 | 0.0121 | 22.0452 | 0.0000 | 13.2921 |
20 | (0.3, 0.7) | (0.9, 0.1) | 0.8427 | 0.4925 | 0.3502 | 0.0121 | 28.8537 | 0.0000 | 16.4328 |
21 | (0.4, 0.6) | (0.5, 0.5) | 0.7973 | 0.7512 | 0.0461 | 0.0121 | 3.8013 | 0.0140 | 1.7153 |
22 | (0.4, 0.6) | (0.6, 0.4) | 0.7973 | 0.6948 | 0.1025 | 0.0121 | 8.4440 | 0.0000 | 4.0142 |
23 | (0.4, 0.6) | (0.7, 0.3) | 0.7973 | 0.6347 | 0.1626 | 0.0121 | 13.3984 | 0.0000 | 6.1125 |
24 | (0.4, 0.6) | (0.8, 0.2) | 0.7973 | 0.5752 | 0.2222 | 0.0121 | 18.3060 | 0.0000 | 9.3551 |
25 | (0.4, 0.6) | (0.9, 0.1) | 0.7973 | 0.4925 | 0.3048 | 0.0121 | 25.1145 | 0.0000 | 12.3113 |
26 | (0.5, 0.5) | (0.6, 0.4) | 0.7512 | 0.6948 | 0.0563 | 0.0121 | 4.6427 | 0.0013 | 2.2713 |
27 | (0.5, 0.5) | (0.7, 0.3) | 0.7512 | 0.6347 | 0.1165 | 0.0121 | 9.5970 | 0.0000 | 4.4953 |
28 | (0.5, 0.5) | (0.8, 0.2) | 0.7512 | 0.5752 | 0.1760 | 0.0121 | 14.5047 | 0.0000 | 7.6636 |
29 | (0.5, 0.5) | (0.9, 0.1) | 0.7512 | 0.4925 | 0.2587 | 0.0121 | 21.3131 | 0.0000 | 10.7722 |
30 | (0.6, 0.4) | (0.7, 0.3) | 0.6948 | 0.6347 | 0.0601 | 0.0121 | 4.9543 | 0.0005 | 2.4554 |
31 | (0.6, 0.4) | (0.8, 0.2) | 0.6948 | 0.5752 | 0.1197 | 0.0121 | 9.8620 | 0.0000 | 5.6052 |
32 | (0.6, 0.4) | (0.9, 0.1) | 0.6948 | 0.4925 | 0.2023 | 0.0121 | 16.6704 | 0.0000 | 9.0039 |
33 | (0.7, 0.3) | (0.8, 0.2) | 0.6347 | 0.5752 | 0.0596 | 0.0121 | 4.9076 | 0.0006 | 2.6325 |
34 | (0.7, 0.3) | (0.9, 0.1) | 0.6347 | 0.4925 | 0.1422 | 0.0121 | 11.7161 | 0.0000 | 6.0041 |
35 | (0.8, 0.2) | (0.9, 0.1) | 0.5752 | 0.4925 | 0.0826 | 0.0121 | 6.8085 | 0.0000 | 4.0457 |
Cost-sensitive sampling for target class balancing
- Under sampling(X)
- Over sampling(O) : a little bit effective
- Combined sampling(X)
test_recall | |
---|---|
sampling_strategy | |
0.0 | 0.303053 |
0.5 | 0.805162 |
0.8 | 0.866887 |
0.9 | 0.882855 |
1.0 | 0.893989 |
F Value | Num DF | Den DF | Pr > F | |
---|---|---|---|---|
sampling_strategy | 15.723825 | 4.0 | 16.0 | 0.000021 |
stat | pval | pval_corr | reject | ||
---|---|---|---|---|---|
group1 | group2 | ||||
0.0 | 0.5 | -2.7108 | 0.0266 | 0.2663 | False |
0.8 | -3.2577 | 0.0116 | 0.1157 | False | |
0.9 | -3.5244 | 0.0078 | 0.078 | False | |
1.0 | -3.7396 | 0.0057 | 0.0571 | False | |
0.5 | 0.8 | -0.3519 | 0.734 | 1.0 | False |
0.9 | -0.4653 | 0.6541 | 1.0 | False | |
1.0 | -0.5531 | 0.5953 | 1.0 | False | |
0.8 | 0.9 | -0.1041 | 0.9197 | 1.0 | False |
1.0 | -0.1851 | 0.8577 | 1.0 | False | |
0.9 | 1.0 | -0.0818 | 0.9368 | 1.0 | False |
Data transformation
- Linear independence of the features: Model Assumption
- Nonlinear transform: Normality; GNB, LDA, QDA
- Linear transform: Standard Scaling (Z-Transform) for LDA
- Constraint: Normalization for LDA, QDA
- Whitening Distribution Outlier
- Robust Scaling / Minmax Scaling, Maxabs Scaling
Linearity effect: effective
test_recall | |
---|---|
treatment | |
_ | 0.3621 |
_H | 0.3621 |
_HV | 0.3849 |
_N | 0.2516 |
_NH | 0.2516 |
_NHV | 0.1976 |
_NV | 0.0000 |
_V | 0.0737 |
sum_sq | df | F | PR(>F) | |
---|---|---|---|---|
C(normality) | 0.1452 | 1.0 | 9.7369 | 0.0038 |
C(heteroscedasticity) | 0.1618 | 1.0 | 10.8528 | 0.0024 |
C(vectorspace) | 0.2039 | 1.0 | 13.6769 | 0.0008 |
C(normality):C(heteroscedasticity) | 0.0081 | 1.0 | 0.5414 | 0.4672 |
C(heteroscedasticity):C(vectorspace) | 0.1618 | 1.0 | 10.8528 | 0.0024 |
C(normality):C(vectorspace) | 0.0010 | 1.0 | 0.0680 | 0.7960 |
C(normality):C(heteroscedasticity):C(vectorspace) | 0.0081 | 1.0 | 0.5414 | 0.4672 |
Residual | 0.4770 | 32.0 | NaN | NaN |
meandiff | p-adj | lower | upper | reject | ||
---|---|---|---|---|---|---|
group1 | group2 | |||||
_N | _NV | -0.2516 | 0.0478 | -0.5018 | -0.0015 | True |
_NH | _NV | -0.2516 | 0.0478 | -0.5018 | -0.0015 | True |
_ | _V | -0.2883 | 0.0149 | -0.5385 | -0.0382 | True |
_H | _V | -0.2883 | 0.0149 | -0.5385 | -0.0382 | True |
_HV | _V | -0.3112 | 0.0069 | -0.5614 | -0.0611 | True |
_ | _NV | -0.3621 | 0.0011 | -0.6122 | -0.1119 | True |
_H | _NV | -0.3621 | 0.0011 | -0.6122 | -0.1119 | True |
_HV | _NV | -0.3849 | 0.0005 | -0.6351 | -0.1348 | True |
_NV | _V | 0.0737 | 0.9776 | -0.1764 | 0.3239 | False |
_ | _HV | 0.0229 | 1.0 | -0.2273 | 0.273 | False |
_H | _HV | 0.0229 | 1.0 | -0.2273 | 0.273 | False |
_ | _H | 0.0 | 1.0 | -0.2501 | 0.2501 | False |
_N | _NH | 0.0 | 1.0 | -0.2501 | 0.2501 | False |
_NHV | -0.0541 | 0.9964 | -0.3042 | 0.1961 | False | |
_NH | _NHV | -0.0541 | 0.9964 | -0.3042 | 0.1961 | False |
_ | _N | -0.1104 | 0.8367 | -0.3606 | 0.1397 | False |
_NH | -0.1104 | 0.8367 | -0.3606 | 0.1397 | False | |
_H | _N | -0.1104 | 0.8367 | -0.3606 | 0.1397 | False |
_NH | -0.1104 | 0.8367 | -0.3606 | 0.1397 | False | |
_NHV | _V | -0.1238 | 0.7446 | -0.374 | 0.1263 | False |
_HV | _N | -0.1333 | 0.671 | -0.3834 | 0.1168 | False |
_NH | -0.1333 | 0.671 | -0.3834 | 0.1168 | False | |
_ | _NHV | -0.1645 | 0.4184 | -0.4146 | 0.0857 | False |
_H | _NHV | -0.1645 | 0.4184 | -0.4146 | 0.0857 | False |
_N | _V | -0.1779 | 0.3224 | -0.4281 | 0.0722 | False |
_NH | _V | -0.1779 | 0.3224 | -0.4281 | 0.0722 | False |
_HV | _NHV | -0.1874 | 0.2634 | -0.4375 | 0.0628 | False |
_NHV | _NV | -0.1976 | 0.2084 | -0.4477 | 0.0526 | False |
Outlier effect: non-effective
test_recall | ||
---|---|---|
scaler | contamination | |
A | 0.00 | 0.260528 |
0.01 | 0.260528 | |
0.05 | 0.260528 | |
0.10 | 0.260528 | |
0.20 | 0.260528 | |
0.50 | 0.260528 | |
M | 0.00 | 0.260528 |
0.01 | 0.260528 | |
0.05 | 0.260528 | |
0.10 | 0.260528 | |
0.20 | 0.260528 | |
0.50 | 0.260528 | |
R | 0.00 | 0.279438 |
0.01 | 0.279438 | |
0.05 | 0.279438 | |
0.10 | 0.279438 | |
0.20 | 0.279438 | |
0.50 | 0.279438 |
sum_sq | df | F | PR(>F) | |
---|---|---|---|---|
C(scaler) | 7.151514e-03 | 2.0 | 1.613890e-01 | 0.851268 |
C(contamination) | 2.223554e-32 | 5.0 | 2.007168e-31 | 1.000000 |
C(scaler):C(contamination) | 1.617393e-31 | 10.0 | 7.299979e-31 | 1.000000 |
Residual | 1.595242e+00 | 72.0 | NaN | NaN |
meandiff | p-adj | lower | upper | reject | ||
---|---|---|---|---|---|---|
group1 | group2 | |||||
A0.0 | R0.0 | 0.0189 | 1.0 | -0.3217 | 0.3595 | False |
R0.01 | 0.0189 | 1.0 | -0.3217 | 0.3595 | False | |
R0.05 | 0.0189 | 1.0 | -0.3217 | 0.3595 | False | |
R0.1 | 0.0189 | 1.0 | -0.3217 | 0.3595 | False | |
R0.2 | 0.0189 | 1.0 | -0.3217 | 0.3595 | False | |
R0.5 | 0.0189 | 1.0 | -0.3217 | 0.3595 | False | |
A0.01 | R0.0 | 0.0189 | 1.0 | -0.3217 | 0.3595 | False |
R0.01 | 0.0189 | 1.0 | -0.3217 | 0.3595 | False | |
R0.05 | 0.0189 | 1.0 | -0.3217 | 0.3595 | False | |
R0.1 | 0.0189 | 1.0 | -0.3217 | 0.3595 | False | |
R0.2 | 0.0189 | 1.0 | -0.3217 | 0.3595 | False | |
R0.5 | 0.0189 | 1.0 | -0.3217 | 0.3595 | False | |
A0.05 | R0.0 | 0.0189 | 1.0 | -0.3217 | 0.3595 | False |
R0.01 | 0.0189 | 1.0 | -0.3217 | 0.3595 | False | |
R0.05 | 0.0189 | 1.0 | -0.3217 | 0.3595 | False | |
R0.1 | 0.0189 | 1.0 | -0.3217 | 0.3595 | False | |
R0.2 | 0.0189 | 1.0 | -0.3217 | 0.3595 | False | |
R0.5 | 0.0189 | 1.0 | -0.3217 | 0.3595 | False | |
A0.1 | R0.0 | 0.0189 | 1.0 | -0.3217 | 0.3595 | False |
R0.01 | 0.0189 | 1.0 | -0.3217 | 0.3595 | False | |
R0.05 | 0.0189 | 1.0 | -0.3217 | 0.3595 | False | |
R0.1 | 0.0189 | 1.0 | -0.3217 | 0.3595 | False | |
R0.2 | 0.0189 | 1.0 | -0.3217 | 0.3595 | False | |
R0.5 | 0.0189 | 1.0 | -0.3217 | 0.3595 | False | |
A0.2 | R0.0 | 0.0189 | 1.0 | -0.3217 | 0.3595 | False |
R0.01 | 0.0189 | 1.0 | -0.3217 | 0.3595 | False | |
R0.05 | 0.0189 | 1.0 | -0.3217 | 0.3595 | False | |
R0.1 | 0.0189 | 1.0 | -0.3217 | 0.3595 | False | |
R0.2 | 0.0189 | 1.0 | -0.3217 | 0.3595 | False | |
R0.5 | 0.0189 | 1.0 | -0.3217 | 0.3595 | False | |
A0.5 | R0.0 | 0.0189 | 1.0 | -0.3217 | 0.3595 | False |
R0.01 | 0.0189 | 1.0 | -0.3217 | 0.3595 | False | |
R0.05 | 0.0189 | 1.0 | -0.3217 | 0.3595 | False | |
R0.1 | 0.0189 | 1.0 | -0.3217 | 0.3595 | False | |
R0.2 | 0.0189 | 1.0 | -0.3217 | 0.3595 | False | |
R0.5 | 0.0189 | 1.0 | -0.3217 | 0.3595 | False | |
M0.0 | R0.0 | 0.0189 | 1.0 | -0.3217 | 0.3595 | False |
R0.01 | 0.0189 | 1.0 | -0.3217 | 0.3595 | False | |
R0.05 | 0.0189 | 1.0 | -0.3217 | 0.3595 | False | |
R0.1 | 0.0189 | 1.0 | -0.3217 | 0.3595 | False | |
R0.2 | 0.0189 | 1.0 | -0.3217 | 0.3595 | False | |
R0.5 | 0.0189 | 1.0 | -0.3217 | 0.3595 | False | |
M0.01 | R0.0 | 0.0189 | 1.0 | -0.3217 | 0.3595 | False |
R0.01 | 0.0189 | 1.0 | -0.3217 | 0.3595 | False | |
R0.05 | 0.0189 | 1.0 | -0.3217 | 0.3595 | False | |
R0.1 | 0.0189 | 1.0 | -0.3217 | 0.3595 | False | |
R0.2 | 0.0189 | 1.0 | -0.3217 | 0.3595 | False | |
R0.5 | 0.0189 | 1.0 | -0.3217 | 0.3595 | False | |
M0.05 | R0.0 | 0.0189 | 1.0 | -0.3217 | 0.3595 | False |
R0.01 | 0.0189 | 1.0 | -0.3217 | 0.3595 | False | |
R0.05 | 0.0189 | 1.0 | -0.3217 | 0.3595 | False | |
R0.1 | 0.0189 | 1.0 | -0.3217 | 0.3595 | False | |
R0.2 | 0.0189 | 1.0 | -0.3217 | 0.3595 | False | |
R0.5 | 0.0189 | 1.0 | -0.3217 | 0.3595 | False | |
M0.1 | R0.0 | 0.0189 | 1.0 | -0.3217 | 0.3595 | False |
R0.01 | 0.0189 | 1.0 | -0.3217 | 0.3595 | False | |
R0.05 | 0.0189 | 1.0 | -0.3217 | 0.3595 | False | |
R0.1 | 0.0189 | 1.0 | -0.3217 | 0.3595 | False | |
R0.2 | 0.0189 | 1.0 | -0.3217 | 0.3595 | False | |
R0.5 | 0.0189 | 1.0 | -0.3217 | 0.3595 | False | |
M0.2 | R0.0 | 0.0189 | 1.0 | -0.3217 | 0.3595 | False |
R0.01 | 0.0189 | 1.0 | -0.3217 | 0.3595 | False | |
R0.05 | 0.0189 | 1.0 | -0.3217 | 0.3595 | False | |
R0.1 | 0.0189 | 1.0 | -0.3217 | 0.3595 | False | |
R0.2 | 0.0189 | 1.0 | -0.3217 | 0.3595 | False | |
R0.5 | 0.0189 | 1.0 | -0.3217 | 0.3595 | False | |
M0.5 | R0.0 | 0.0189 | 1.0 | -0.3217 | 0.3595 | False |
R0.01 | 0.0189 | 1.0 | -0.3217 | 0.3595 | False | |
R0.05 | 0.0189 | 1.0 | -0.3217 | 0.3595 | False | |
R0.1 | 0.0189 | 1.0 | -0.3217 | 0.3595 | False | |
R0.2 | 0.0189 | 1.0 | -0.3217 | 0.3595 | False | |
R0.5 | 0.0189 | 1.0 | -0.3217 | 0.3595 | False | |
A0.0 | A0.01 | 0.0 | 1.0 | -0.3406 | 0.3406 | False |
A0.05 | 0.0 | 1.0 | -0.3406 | 0.3406 | False | |
A0.1 | 0.0 | 1.0 | -0.3406 | 0.3406 | False | |
A0.2 | 0.0 | 1.0 | -0.3406 | 0.3406 | False | |
A0.5 | 0.0 | 1.0 | -0.3406 | 0.3406 | False | |
M0.0 | 0.0 | 1.0 | -0.3406 | 0.3406 | False | |
M0.01 | 0.0 | 1.0 | -0.3406 | 0.3406 | False | |
M0.05 | 0.0 | 1.0 | -0.3406 | 0.3406 | False | |
M0.1 | 0.0 | 1.0 | -0.3406 | 0.3406 | False | |
M0.2 | 0.0 | 1.0 | -0.3406 | 0.3406 | False | |
M0.5 | 0.0 | 1.0 | -0.3406 | 0.3406 | False | |
A0.01 | A0.05 | 0.0 | 1.0 | -0.3406 | 0.3406 | False |
A0.1 | 0.0 | 1.0 | -0.3406 | 0.3406 | False | |
A0.2 | 0.0 | 1.0 | -0.3406 | 0.3406 | False | |
A0.5 | 0.0 | 1.0 | -0.3406 | 0.3406 | False | |
M0.0 | 0.0 | 1.0 | -0.3406 | 0.3406 | False | |
M0.01 | 0.0 | 1.0 | -0.3406 | 0.3406 | False | |
M0.05 | 0.0 | 1.0 | -0.3406 | 0.3406 | False | |
M0.1 | 0.0 | 1.0 | -0.3406 | 0.3406 | False | |
M0.2 | 0.0 | 1.0 | -0.3406 | 0.3406 | False | |
M0.5 | 0.0 | 1.0 | -0.3406 | 0.3406 | False | |
A0.05 | A0.1 | 0.0 | 1.0 | -0.3406 | 0.3406 | False |
A0.2 | 0.0 | 1.0 | -0.3406 | 0.3406 | False | |
A0.5 | 0.0 | 1.0 | -0.3406 | 0.3406 | False | |
M0.0 | 0.0 | 1.0 | -0.3406 | 0.3406 | False | |
M0.01 | 0.0 | 1.0 | -0.3406 | 0.3406 | False | |
M0.05 | 0.0 | 1.0 | -0.3406 | 0.3406 | False | |
M0.1 | 0.0 | 1.0 | -0.3406 | 0.3406 | False | |
M0.2 | 0.0 | 1.0 | -0.3406 | 0.3406 | False | |
M0.5 | 0.0 | 1.0 | -0.3406 | 0.3406 | False | |
A0.1 | A0.2 | 0.0 | 1.0 | -0.3406 | 0.3406 | False |
A0.5 | 0.0 | 1.0 | -0.3406 | 0.3406 | False | |
M0.0 | 0.0 | 1.0 | -0.3406 | 0.3406 | False | |
M0.01 | 0.0 | 1.0 | -0.3406 | 0.3406 | False | |
M0.05 | 0.0 | 1.0 | -0.3406 | 0.3406 | False | |
M0.1 | 0.0 | 1.0 | -0.3406 | 0.3406 | False | |
M0.2 | 0.0 | 1.0 | -0.3406 | 0.3406 | False | |
M0.5 | 0.0 | 1.0 | -0.3406 | 0.3406 | False | |
A0.2 | A0.5 | 0.0 | 1.0 | -0.3406 | 0.3406 | False |
M0.0 | 0.0 | 1.0 | -0.3406 | 0.3406 | False | |
M0.01 | 0.0 | 1.0 | -0.3406 | 0.3406 | False | |
M0.05 | 0.0 | 1.0 | -0.3406 | 0.3406 | False | |
M0.1 | 0.0 | 1.0 | -0.3406 | 0.3406 | False | |
M0.2 | 0.0 | 1.0 | -0.3406 | 0.3406 | False | |
M0.5 | 0.0 | 1.0 | -0.3406 | 0.3406 | False | |
A0.5 | M0.0 | 0.0 | 1.0 | -0.3406 | 0.3406 | False |
M0.01 | 0.0 | 1.0 | -0.3406 | 0.3406 | False | |
M0.05 | 0.0 | 1.0 | -0.3406 | 0.3406 | False | |
M0.1 | 0.0 | 1.0 | -0.3406 | 0.3406 | False | |
M0.2 | 0.0 | 1.0 | -0.3406 | 0.3406 | False | |
M0.5 | 0.0 | 1.0 | -0.3406 | 0.3406 | False | |
M0.0 | M0.01 | 0.0 | 1.0 | -0.3406 | 0.3406 | False |
M0.05 | 0.0 | 1.0 | -0.3406 | 0.3406 | False | |
M0.1 | 0.0 | 1.0 | -0.3406 | 0.3406 | False | |
M0.2 | 0.0 | 1.0 | -0.3406 | 0.3406 | False | |
M0.5 | 0.0 | 1.0 | -0.3406 | 0.3406 | False | |
M0.01 | M0.05 | 0.0 | 1.0 | -0.3406 | 0.3406 | False |
M0.1 | 0.0 | 1.0 | -0.3406 | 0.3406 | False | |
M0.2 | 0.0 | 1.0 | -0.3406 | 0.3406 | False | |
M0.5 | 0.0 | 1.0 | -0.3406 | 0.3406 | False | |
M0.05 | M0.1 | 0.0 | 1.0 | -0.3406 | 0.3406 | False |
M0.2 | 0.0 | 1.0 | -0.3406 | 0.3406 | False | |
M0.5 | 0.0 | 1.0 | -0.3406 | 0.3406 | False | |
M0.1 | M0.2 | 0.0 | 1.0 | -0.3406 | 0.3406 | False |
M0.5 | 0.0 | 1.0 | -0.3406 | 0.3406 | False | |
M0.2 | M0.5 | 0.0 | 1.0 | -0.3406 | 0.3406 | False |
R0.0 | R0.01 | 0.0 | 1.0 | -0.3406 | 0.3406 | False |
R0.05 | 0.0 | 1.0 | -0.3406 | 0.3406 | False | |
R0.1 | 0.0 | 1.0 | -0.3406 | 0.3406 | False | |
R0.2 | 0.0 | 1.0 | -0.3406 | 0.3406 | False | |
R0.5 | 0.0 | 1.0 | -0.3406 | 0.3406 | False | |
R0.01 | R0.05 | 0.0 | 1.0 | -0.3406 | 0.3406 | False |
R0.1 | 0.0 | 1.0 | -0.3406 | 0.3406 | False | |
R0.2 | 0.0 | 1.0 | -0.3406 | 0.3406 | False | |
R0.5 | 0.0 | 1.0 | -0.3406 | 0.3406 | False | |
R0.05 | R0.1 | 0.0 | 1.0 | -0.3406 | 0.3406 | False |
R0.2 | 0.0 | 1.0 | -0.3406 | 0.3406 | False | |
R0.5 | 0.0 | 1.0 | -0.3406 | 0.3406 | False | |
R0.1 | R0.2 | 0.0 | 1.0 | -0.3406 | 0.3406 | False |
R0.5 | 0.0 | 1.0 | -0.3406 | 0.3406 | False | |
R0.2 | R0.5 | 0.0 | 1.0 | -0.3406 | 0.3406 | False |
Linear relationship analysis
Regression Analysis
(Note) Regression analysis has been conducted with one-hot encoding for categorical variables.
Logit analysis summary table
- representative positive effect factors on deposit: C(poutcome)[T.success], C(month)[T.mar], C(job)[T.student], duration
- representative negative effect factors on deposit: C(contact)[unknown], C(contact)[telephone], C(contact)[cellular], C(month)[T.jan], campaign
with feature interaction
centering | standardizing | |||||||||||||
---|---|---|---|---|---|---|---|---|---|---|---|---|---|---|
feature | coef | std err | z | P>|z| | [0.025 | 0.975] | feature | coef | std err | z | P>|z| | [0.025 | 0.975] | |
1 | C(contact)[cellular] | -1.5389 | 0.136 | -11.350 | 0.000 | -1.805 | -1.273 | C(contact)[cellular] | -1.5389 | 0.136 | -11.350 | 0.000 | -1.805 | -1.273 |
2 | C(contact)[telephone] | -1.7394 | 0.153 | -11.356 | 0.000 | -2.040 | -1.439 | C(contact)[telephone] | -1.7394 | 0.153 | -11.356 | 0.000 | -2.040 | -1.439 |
3 | C(contact)[unknown] | -3.2081 | 0.157 | -20.396 | 0.000 | -3.516 | -2.900 | C(contact)[unknown] | -3.2081 | 0.157 | -20.396 | 0.000 | -3.516 | -2.900 |
4 | C(housing)[T.yes] | -0.6947 | 0.044 | -15.956 | 0.000 | -0.780 | -0.609 | C(housing)[T.yes] | -0.6947 | 0.044 | -15.956 | 0.000 | -0.780 | -0.609 |
5 | C(job)[T.blue-collar] | -0.4229 | 0.070 | -6.069 | 0.000 | -0.559 | -0.286 | C(job)[T.blue-collar] | -0.4229 | 0.070 | -6.069 | 0.000 | -0.559 | -0.286 |
6 | C(job)[T.entrepreneur] | -0.3586 | 0.123 | -2.912 | 0.004 | -0.600 | -0.117 | C(job)[T.entrepreneur] | -0.3586 | 0.123 | -2.912 | 0.004 | -0.600 | -0.117 |
7 | C(job)[T.housemaid] | -0.6010 | 0.133 | -4.527 | 0.000 | -0.861 | -0.341 | C(job)[T.housemaid] | -0.6010 | 0.133 | -4.527 | 0.000 | -0.861 | -0.341 |
8 | C(job)[T.management] | -0.0188 | 0.064 | -0.295 | 0.768 | -0.144 | 0.106 | C(job)[T.management] | -0.0188 | 0.064 | -0.295 | 0.768 | -0.144 | 0.106 |
9 | C(job)[T.retired] | 0.1480 | 0.084 | 1.762 | 0.078 | -0.017 | 0.313 | C(job)[T.retired] | 0.1480 | 0.084 | 1.762 | 0.078 | -0.017 | 0.313 |
10 | C(job)[T.self-employed] | -0.2023 | 0.109 | -1.864 | 0.062 | -0.415 | 0.010 | C(job)[T.self-employed] | -0.2023 | 0.109 | -1.864 | 0.062 | -0.415 | 0.010 |
11 | C(job)[T.services] | -0.2544 | 0.084 | -3.037 | 0.002 | -0.419 | -0.090 | C(job)[T.services] | -0.2544 | 0.084 | -3.037 | 0.002 | -0.419 | -0.090 |
12 | C(job)[T.student] | 0.5845 | 0.104 | 5.638 | 0.000 | 0.381 | 0.788 | C(job)[T.student] | 0.5845 | 0.104 | 5.638 | 0.000 | 0.381 | 0.788 |
13 | C(job)[T.technician] | -0.1352 | 0.068 | -1.981 | 0.048 | -0.269 | -0.001 | C(job)[T.technician] | -0.1352 | 0.068 | -1.981 | 0.048 | -0.269 | -0.001 |
14 | C(job)[T.unemployed] | -0.1438 | 0.110 | -1.304 | 0.192 | -0.360 | 0.072 | C(job)[T.unemployed] | -0.1438 | 0.110 | -1.304 | 0.192 | -0.360 | 0.072 |
15 | C(job)[T.unknown] | -0.2998 | 0.230 | -1.302 | 0.193 | -0.751 | 0.151 | C(job)[T.unknown] | -0.2998 | 0.230 | -1.302 | 0.193 | -0.751 | 0.151 |
16 | C(month)[T.aug] | -0.7064 | 0.078 | -9.036 | 0.000 | -0.860 | -0.553 | C(month)[T.aug] | -0.7064 | 0.078 | -9.036 | 0.000 | -0.860 | -0.553 |
17 | C(month)[T.dec] | 0.7147 | 0.176 | 4.053 | 0.000 | 0.369 | 1.060 | C(month)[T.dec] | 0.7147 | 0.176 | 4.053 | 0.000 | 0.369 | 1.060 |
18 | C(month)[T.feb] | -0.1413 | 0.089 | -1.583 | 0.113 | -0.316 | 0.034 | C(month)[T.feb] | -0.1413 | 0.089 | -1.583 | 0.113 | -0.316 | 0.034 |
19 | C(month)[T.jan] | -1.2649 | 0.121 | -10.412 | 0.000 | -1.503 | -1.027 | C(month)[T.jan] | -1.2649 | 0.121 | -10.412 | 0.000 | -1.503 | -1.027 |
20 | C(month)[T.jul] | -0.9189 | 0.077 | -11.995 | 0.000 | -1.069 | -0.769 | C(month)[T.jul] | -0.9189 | 0.077 | -11.995 | 0.000 | -1.069 | -0.769 |
21 | C(month)[T.jun] | 0.4662 | 0.094 | 4.980 | 0.000 | 0.283 | 0.650 | C(month)[T.jun] | 0.4662 | 0.094 | 4.980 | 0.000 | 0.283 | 0.650 |
22 | C(month)[T.mar] | 1.6243 | 0.119 | 13.595 | 0.000 | 1.390 | 1.858 | C(month)[T.mar] | 1.6243 | 0.119 | 13.595 | 0.000 | 1.390 | 1.858 |
23 | C(month)[T.may] | -0.3804 | 0.072 | -5.281 | 0.000 | -0.522 | -0.239 | C(month)[T.may] | -0.3804 | 0.072 | -5.281 | 0.000 | -0.522 | -0.239 |
24 | C(month)[T.nov] | -0.9173 | 0.084 | -10.905 | 0.000 | -1.082 | -0.752 | C(month)[T.nov] | -0.9173 | 0.084 | -10.905 | 0.000 | -1.082 | -0.752 |
25 | C(month)[T.oct] | 0.8956 | 0.108 | 8.293 | 0.000 | 0.684 | 1.107 | C(month)[T.oct] | 0.8956 | 0.108 | 8.293 | 0.000 | 0.684 | 1.107 |
26 | C(month)[T.sep] | 0.8829 | 0.119 | 7.392 | 0.000 | 0.649 | 1.117 | C(month)[T.sep] | 0.8829 | 0.119 | 7.392 | 0.000 | 0.649 | 1.117 |
27 | C(poutcome)[T.other] | 0.3333 | 0.169 | 1.970 | 0.049 | 0.002 | 0.665 | C(poutcome)[T.other] | 0.3333 | 0.169 | 1.970 | 0.049 | 0.002 | 0.665 |
28 | C(poutcome)[T.success] | 2.4414 | 0.160 | 15.269 | 0.000 | 2.128 | 2.755 | C(poutcome)[T.success] | 2.4414 | 0.160 | 15.269 | 0.000 | 2.128 | 2.755 |
29 | C(poutcome)[T.unknown] | -0.0414 | 0.227 | -0.183 | 0.855 | -0.486 | 0.403 | C(poutcome)[T.unknown] | -0.0414 | 0.227 | -0.183 | 0.855 | -0.486 | 0.403 |
30 | balance | 1.525e-05 | 5.09e-06 | 2.996 | 0.003 | 5.27e-06 | 2.52e-05 | balance | 0.0464 | 0.015 | 2.996 | 0.003 | 0.016 | 0.077 |
31 | day | 0.0107 | 0.002 | 4.281 | 0.000 | 0.006 | 0.016 | day | 0.0889 | 0.021 | 4.281 | 0.000 | 0.048 | 0.130 |
32 | duration | 0.0042 | 6.42e-05 | 65.169 | 0.000 | 0.004 | 0.004 | duration | 1.0783 | 0.017 | 65.169 | 0.000 | 1.046 | 1.111 |
33 | campaign | -0.0940 | 0.010 | -9.206 | 0.000 | -0.114 | -0.074 | campaign | -0.2912 | 0.032 | -9.206 | 0.000 | -0.353 | -0.229 |
34 | pdays | 0.0002 | 0.000 | 0.413 | 0.679 | -0.001 | 0.001 | pdays | 0.0190 | 0.046 | 0.413 | 0.679 | -0.071 | 0.109 |
35 | pdays:C(poutcome)[T.other] | -0.0003 | 0.001 | -0.359 | 0.719 | -0.002 | 0.001 | pdays:C(poutcome)[T.other] | -0.0266 | 0.074 | -0.359 | 0.719 | -0.172 | 0.119 |
36 | pdays:C(poutcome)[T.success] | -0.0004 | 0.001 | -0.584 | 0.560 | -0.002 | 0.001 | pdays:C(poutcome)[T.success] | -0.0444 | 0.076 | -0.584 | 0.560 | -0.194 | 0.105 |
37 | pdays:C(poutcome)[T.unknown] | 0.0041 | 0.008 | 0.523 | 0.601 | -0.011 | 0.020 | pdays:C(poutcome)[T.unknown] | 0.4130 | 0.790 | 0.523 | 0.601 | -1.136 | 1.962 |
38 | previous | 0.0416 | 0.021 | 1.945 | 0.052 | -0.000 | 0.084 | previous | 0.0959 | 0.049 | 1.945 | 0.052 | -0.001 | 0.193 |
39 | previous:C(poutcome)[T.other] | -0.0269 | 0.017 | -1.610 | 0.107 | -0.060 | 0.006 | previous:C(poutcome)[T.other] | -0.0621 | 0.039 | -1.610 | 0.107 | -0.138 | 0.013 |
40 | previous:C(poutcome)[T.success] | -0.0179 | 0.030 | -0.606 | 0.544 | -0.076 | 0.040 | previous:C(poutcome)[T.success] | -0.0413 | 0.068 | -0.606 | 0.544 | -0.175 | 0.092 |
41 | previous:C(poutcome)[T.unknown] | -0.4841 | 0.694 | -0.697 | 0.486 | -1.844 | 0.876 | previous:C(poutcome)[T.unknown] | -1.1150 | 1.599 | -0.697 | 0.486 | -4.248 | 2.018 |
42 | pdays:previous | -4.709e-05 | 7.42e-05 | -0.634 | 0.526 | -0.000 | 9.84e-05 | pdays:previous | -0.0109 | 0.017 | -0.634 | 0.526 | -0.044 | 0.023 |
Variance inflation factors
features with multi-collinearity (vif > 10): default, marital, loan, education, age
feature | variance inflation factor without target | variance inflation factor with target | ranking |
default | 89.590922 | 90.757180 | 1.0 |
marital | 34.094449 | 34.095701 | 2.0 |
loan | 29.192732 | 29.204954 | 3.0 |
education | 27.754247 | 27.754449 | 4.0 |
age | 18.582507 | 18.598972 | 5.0 |
job | 9.713535 | 9.744778 | 6.0 |
housing | 9.418423 | 9.458427 | 7.0 |
contact | 8.074904 | 8.092028 | 8.0 |
day | 4.757858 | 4.758009 | 9.0 |
month | 3.446856 | 3.559682 | 10.0 |
poutcome | 2.899358 | 3.101371 | 11.0 |
duration | 2.022798 | 2.419260 | 12.0 |
campaign | 1.873813 | 1.874478 | 13.0 |
pdays | 1.721085 | 1.721512 | 14.0 |
y | - | 1.597047 | 15.0 |
previous | 1.373109 | 1.373142 | 16.0 |
balance | 1.228526 | 1.229092 | 17.0 |
Covariance Analysis
As shown in the heatmap, "deposit yes" exhibits strong correlations with customer attributes. I have performed regression analysis and principle component analysis(PCA) to explore impact of individual attributes on "deposit yes".
Principle component analysis
- Features with high-variance : balance, age, day, duration, campaign, pdays, previous
- Selected strongly correlated features
- Correlation between explainatory features
- positive correlation: (job, education), (pdays, previous), (pdays, poutcome), (previous, poutcome)
- negative correlation: (age, marital)
- Target feature correlation
- positive correlation: duration, poutcome, month
- Correlation between explainatory features
- Efficient feature dimension range: 7 ~ 9
Regression coefficient without feature interaction
(Note) Covariance analysis has been conducted without one-hot encoding for categorical variables.
0 | 1 | 2 | 3 | 4 | 5 | 6 | 7 | 8 | 9 | 10 | 11 | 12 | 13 | 14 | 15 | |
centering | -0.0 | 4.0 | 4.0 | 3.0 | 0.0 | 0.0 | 6.0 | 4.0 | 7.0 | -0.0 | 4.0 | 0.0 | -0.0 | 0.0 | 0.0 | 4.0 |
standardizing | -0.0 | 0.0 | 0.0 | 0.0 | 0.0 | 0.0 | 0.0 | 0.0 | 0.0 | -0.0 | 0.0 | 1.0 | -0.0 | 0.0 | 0.0 | 0.0 |
pc_centering | 0.0 | 0.0 | 0.0 | 0.0 | -0.0 | -0.0 | 0.0 | -8.0 | 4.0 | -9.0 | -0.0 | 0.0 | -4.0 | -2.0 | 2.0 | -0.0 |
pc_standardizing | 1.0 | -0.0 | -0.0 | -0.0 | -0.0 | 1.0 | -0.0 | -0.0 | -0.0 | 0.0 | -0.0 | -0.0 | -0.0 | -0.0 | 0.0 | 0.0 |
centering & standardizing : '0:age', '1:job', '2:marital', '3:education', '4:default', '5:balance', '6:housing', '7:loan', '8:contact', '9:day', '10:month', '11:duration', '12:campaign', '13:pdays', '14:previous', '15:poutcome'
Exploratory factor analysis
: (orthogonal) varimax rotation
Exploratory Data Analysis
Exploration of association with target feature: multivariate analysis
y | Level0 | duration | Level1 | poutcome | Level2 | |||||||
---|---|---|---|---|---|---|---|---|---|---|---|---|
count | probaility | rank | count | probaility | rank | count | probaility | rank | ||||
0 | no | 39922 | 0.883015 | 1.0 | (-4.918, 491.8] | 36702 | 0.811794 | 1.0 | failure | 3990 | 0.088253 | 2.0 |
1 | no | 39922 | 0.883015 | 1.0 | (-4.918, 491.8] | 36702 | 0.811794 | 1.0 | other | 1407 | 0.031121 | 5.0 |
2 | no | 39922 | 0.883015 | 1.0 | (-4.918, 491.8] | 36702 | 0.811794 | 1.0 | success | 486 | 0.010750 | 8.0 |
3 | no | 39922 | 0.883015 | 1.0 | (-4.918, 491.8] | 36702 | 0.811794 | 1.0 | unknown | 30819 | 0.681670 | 1.0 |
4 | no | 39922 | 0.883015 | 1.0 | (1475.4, 1967.2] | 64 | 0.001416 | 8.0 | failure | 6 | 0.000133 | 31.5 |
5 | no | 39922 | 0.883015 | 1.0 | (1475.4, 1967.2] | 64 | 0.001416 | 8.0 | other | 3 | 0.000066 | 37.0 |
6 | no | 39922 | 0.883015 | 1.0 | (1475.4, 1967.2] | 64 | 0.001416 | 8.0 | success | 1 | 0.000022 | 42.0 |
7 | no | 39922 | 0.883015 | 1.0 | (1475.4, 1967.2] | 64 | 0.001416 | 8.0 | unknown | 54 | 0.001194 | 19.0 |
8 | no | 39922 | 0.883015 | 1.0 | (1967.2, 2459.0] | 20 | 0.000442 | 10.0 | failure | 2 | 0.000044 | 38.0 |
9 | no | 39922 | 0.883015 | 1.0 | (1967.2, 2459.0] | 20 | 0.000442 | 10.0 | other | 1 | 0.000022 | 42.0 |
10 | no | 39922 | 0.883015 | 1.0 | (1967.2, 2459.0] | 20 | 0.000442 | 10.0 | unknown | 17 | 0.000376 | 27.0 |
11 | no | 39922 | 0.883015 | 1.0 | (2459.0, 2950.8] | 4 | 0.000088 | 14.0 | unknown | 4 | 0.000088 | 35.5 |
12 | no | 39922 | 0.883015 | 1.0 | (2950.8, 3442.6] | 6 | 0.000133 | 12.0 | unknown | 6 | 0.000133 | 31.5 |
13 | no | 39922 | 0.883015 | 1.0 | (3442.6, 3934.4] | 1 | 0.000022 | 16.0 | unknown | 1 | 0.000022 | 42.0 |
14 | no | 39922 | 0.883015 | 1.0 | (4426.2, 4918.0] | 1 | 0.000022 | 16.0 | unknown | 1 | 0.000022 | 42.0 |
15 | no | 39922 | 0.883015 | 1.0 | (491.8, 983.6] | 2776 | 0.061401 | 3.0 | failure | 249 | 0.005508 | 12.0 |
16 | no | 39922 | 0.883015 | 1.0 | (491.8, 983.6] | 2776 | 0.061401 | 3.0 | other | 104 | 0.002300 | 16.0 |
17 | no | 39922 | 0.883015 | 1.0 | (491.8, 983.6] | 2776 | 0.061401 | 3.0 | success | 39 | 0.000863 | 20.0 |
18 | no | 39922 | 0.883015 | 1.0 | (491.8, 983.6] | 2776 | 0.061401 | 3.0 | unknown | 2384 | 0.052731 | 3.0 |
19 | no | 39922 | 0.883015 | 1.0 | (983.6, 1475.4] | 348 | 0.007697 | 6.0 | failure | 36 | 0.000796 | 21.0 |
20 | no | 39922 | 0.883015 | 1.0 | (983.6, 1475.4] | 348 | 0.007697 | 6.0 | other | 18 | 0.000398 | 26.0 |
21 | no | 39922 | 0.883015 | 1.0 | (983.6, 1475.4] | 348 | 0.007697 | 6.0 | success | 7 | 0.000155 | 29.5 |
22 | no | 39922 | 0.883015 | 1.0 | (983.6, 1475.4] | 348 | 0.007697 | 6.0 | unknown | 287 | 0.006348 | 11.0 |
23 | yes | 5289 | 0.116985 | 2.0 | (-4.918, 491.8] | 2975 | 0.065803 | 2.0 | failure | 406 | 0.008980 | 10.0 |
24 | yes | 5289 | 0.116985 | 2.0 | (-4.918, 491.8] | 2975 | 0.065803 | 2.0 | other | 208 | 0.004601 | 13.0 |
25 | yes | 5289 | 0.116985 | 2.0 | (-4.918, 491.8] | 2975 | 0.065803 | 2.0 | success | 782 | 0.017297 | 7.0 |
26 | yes | 5289 | 0.116985 | 2.0 | (-4.918, 491.8] | 2975 | 0.065803 | 2.0 | unknown | 1579 | 0.034925 | 4.0 |
27 | yes | 5289 | 0.116985 | 2.0 | (1475.4, 1967.2] | 112 | 0.002477 | 7.0 | failure | 9 | 0.000199 | 28.0 |
28 | yes | 5289 | 0.116985 | 2.0 | (1475.4, 1967.2] | 112 | 0.002477 | 7.0 | other | 4 | 0.000088 | 35.5 |
29 | yes | 5289 | 0.116985 | 2.0 | (1475.4, 1967.2] | 112 | 0.002477 | 7.0 | success | 5 | 0.000111 | 33.5 |
30 | yes | 5289 | 0.116985 | 2.0 | (1475.4, 1967.2] | 112 | 0.002477 | 7.0 | unknown | 94 | 0.002079 | 17.0 |
31 | yes | 5289 | 0.116985 | 2.0 | (1967.2, 2459.0] | 23 | 0.000509 | 9.0 | failure | 1 | 0.000022 | 42.0 |
32 | yes | 5289 | 0.116985 | 2.0 | (1967.2, 2459.0] | 23 | 0.000509 | 9.0 | success | 1 | 0.000022 | 42.0 |
33 | yes | 5289 | 0.116985 | 2.0 | (1967.2, 2459.0] | 23 | 0.000509 | 9.0 | unknown | 21 | 0.000464 | 24.0 |
34 | yes | 5289 | 0.116985 | 2.0 | (2459.0, 2950.8] | 7 | 0.000155 | 11.0 | unknown | 7 | 0.000155 | 29.5 |
35 | yes | 5289 | 0.116985 | 2.0 | (2950.8, 3442.6] | 5 | 0.000111 | 13.0 | unknown | 5 | 0.000111 | 33.5 |
36 | yes | 5289 | 0.116985 | 2.0 | (3442.6, 3934.4] | 1 | 0.000022 | 16.0 | unknown | 1 | 0.000022 | 42.0 |
37 | yes | 5289 | 0.116985 | 2.0 | (491.8, 983.6] | 1649 | 0.036473 | 4.0 | failure | 170 | 0.003760 | 14.0 |
38 | yes | 5289 | 0.116985 | 2.0 | (491.8, 983.6] | 1649 | 0.036473 | 4.0 | other | 76 | 0.001681 | 18.0 |
39 | yes | 5289 | 0.116985 | 2.0 | (491.8, 983.6] | 1649 | 0.036473 | 4.0 | success | 167 | 0.003694 | 15.0 |
40 | yes | 5289 | 0.116985 | 2.0 | (491.8, 983.6] | 1649 | 0.036473 | 4.0 | unknown | 1236 | 0.027338 | 6.0 |
41 | yes | 5289 | 0.116985 | 2.0 | (983.6, 1475.4] | 517 | 0.011435 | 5.0 | failure | 32 | 0.000708 | 22.0 |
42 | yes | 5289 | 0.116985 | 2.0 | (983.6, 1475.4] | 517 | 0.011435 | 5.0 | other | 19 | 0.000420 | 25.0 |
43 | yes | 5289 | 0.116985 | 2.0 | (983.6, 1475.4] | 517 | 0.011435 | 5.0 | success | 23 | 0.000509 | 23.0 |
44 | yes | 5289 | 0.116985 | 2.0 | (983.6, 1475.4] | 517 | 0.011435 | 5.0 | unknown | 443 | 0.009799 | 9.0 |
support | confidence | lift | ||||||
---|---|---|---|---|---|---|---|---|
CondFreq | no | yes | no | yes | no | yes | ||
duration | poutcome | |||||||
(-4.918, 491.8] | unknown | 32398.0 | 30819.0 | 1579.0 | 0.951262 | 0.048738 | 1.077289 | 0.416615 |
failure | 4396.0 | 3990.0 | 406.0 | 0.907643 | 0.092357 | 1.027891 | 0.789476 | |
other | 1615.0 | 1407.0 | 208.0 | 0.871207 | 0.128793 | 0.986628 | 1.100934 | |
success | 1268.0 | 486.0 | 782.0 | 0.383281 | 0.616719 | 0.434059 | 5.271789 | |
(491.8, 983.6] | unknown | 3620.0 | 2384.0 | 1236.0 | 0.658564 | 0.341436 | 0.745812 | 2.918639 |
failure | 419.0 | 249.0 | 170.0 | 0.594272 | 0.405728 | 0.673003 | 3.468210 | |
other | 180.0 | 104.0 | 76.0 | 0.577778 | 0.422222 | 0.654324 | 3.609206 | |
success | 206.0 | 39.0 | 167.0 | 0.189320 | 0.810680 | 0.214402 | 6.929786 | |
(1475.4, 1967.2] | unknown | 148.0 | 54.0 | 94.0 | 0.364865 | 0.635135 | 0.413203 | 5.429211 |
failure | 15.0 | 6.0 | 9.0 | 0.400000 | 0.600000 | 0.452993 | 5.128871 | |
other | 7.0 | 3.0 | 4.0 | 0.428571 | 0.571429 | 0.485350 | 4.884639 | |
success | 6.0 | 1.0 | 5.0 | 0.166667 | 0.833333 | 0.188747 | 7.123432 | |
(983.6, 1475.4] | unknown | 730.0 | 287.0 | 443.0 | 0.393151 | 0.606849 | 0.445237 | 5.187420 |
failure | 68.0 | 36.0 | 32.0 | 0.529412 | 0.470588 | 0.599550 | 4.022644 | |
other | 37.0 | 18.0 | 19.0 | 0.486486 | 0.513514 | 0.550938 | 4.389574 | |
success | 30.0 | 7.0 | 23.0 | 0.233333 | 0.766667 | 0.264246 | 6.553558 | |
(1967.2, 2459.0] | unknown | 38.0 | 17.0 | 21.0 | 0.447368 | 0.552632 | 0.506637 | 4.723960 |
failure | 3.0 | 2.0 | 1.0 | 0.666667 | 0.333333 | 0.754989 | 2.849373 | |
other | 1.0 | 1.0 | 0.0 | 1.000000 | 0.000000 | 1.132483 | 0.000000 | |
success | 1.0 | 0.0 | 1.0 | 0.000000 | 1.000000 | 0.000000 | 8.548119 | |
(2459.0, 2950.8] | unknown | 11.0 | 4.0 | 7.0 | 0.363636 | 0.636364 | 0.411812 | 5.439712 |
failure | 0.0 | 0.0 | 0.0 | 0.000000 | 0.000000 | NaN | NaN | |
other | 0.0 | 0.0 | 0.0 | 0.000000 | 0.000000 | NaN | NaN | |
success | 0.0 | 0.0 | 0.0 | 0.000000 | 0.000000 | NaN | NaN | |
(2950.8, 3442.6] | unknown | 11.0 | 6.0 | 5.0 | 0.545455 | 0.454545 | 0.617718 | 3.885509 |
failure | 0.0 | 0.0 | 0.0 | 0.000000 | 0.000000 | NaN | NaN | |
other | 0.0 | 0.0 | 0.0 | 0.000000 | 0.000000 | NaN | NaN | |
success | 0.0 | 0.0 | 0.0 | 0.000000 | 0.000000 | NaN | NaN | |
(3442.6, 3934.4] | unknown | 2.0 | 1.0 | 1.0 | 0.500000 | 0.500000 | 0.566242 | 4.274059 |
failure | 0.0 | 0.0 | 0.0 | 0.000000 | 0.000000 | NaN | NaN | |
other | 0.0 | 0.0 | 0.0 | 0.000000 | 0.000000 | NaN | NaN | |
success | 0.0 | 0.0 | 0.0 | 0.000000 | 0.000000 | NaN | NaN | |
(4426.2, 4918.0] | unknown | 1.0 | 1.0 | 0.0 | 1.000000 | 0.000000 | 1.132483 | 0.000000 |
failure | 0.0 | 0.0 | 0.0 | 0.000000 | 0.000000 | NaN | NaN | |
other | 0.0 | 0.0 | 0.0 | 0.000000 | 0.000000 | NaN | NaN | |
success | 0.0 | 0.0 | 0.0 | 0.000000 | 0.000000 | NaN | NaN |
Feature importance and information values for target variable
feature | feature importance | fi ranking | information values | iv ranking |
duration | 0.297104 | 1.0 | 1.816187 | 1 |
balance | 0.120111 | 2.0 | 1.280381 | 2 |
poutcome | 0.098661 | 3.0 | 0.514609 | 4 |
month | 0.091646 | 4.0 | 0.436131 | 5 |
age | 0.091354 | 5.0 | 0.226923 | 8 |
day | 0.084840 | 6.0 | 0.117758 | 11 |
job | 0.045463 | 7.0 | 0.155697 | 10 |
pdays | 0.036583 | 8.0 | 0.559210 | 3 |
campaign | 0.033835 | 9.0 | 0.089986 | 12 |
contact | 0.023641 | 10.0 | 0.300396 | 6 |
education | 0.020378 | 11.0 | 0.050112 | 14 |
marital | 0.016275 | 12.0 | 0.040127 | 15 |
previous | 0.016039 | 13.0 | 0.230969 | 7 |
housing | 0.015632 | 14.0 | 0.188681 | 9 |
loan | 0.007100 | 15.0 | 0.054859 | 13 |
default | 0.001339 | 16.0 | 0.006256 | 16 |
Decision path about target variable
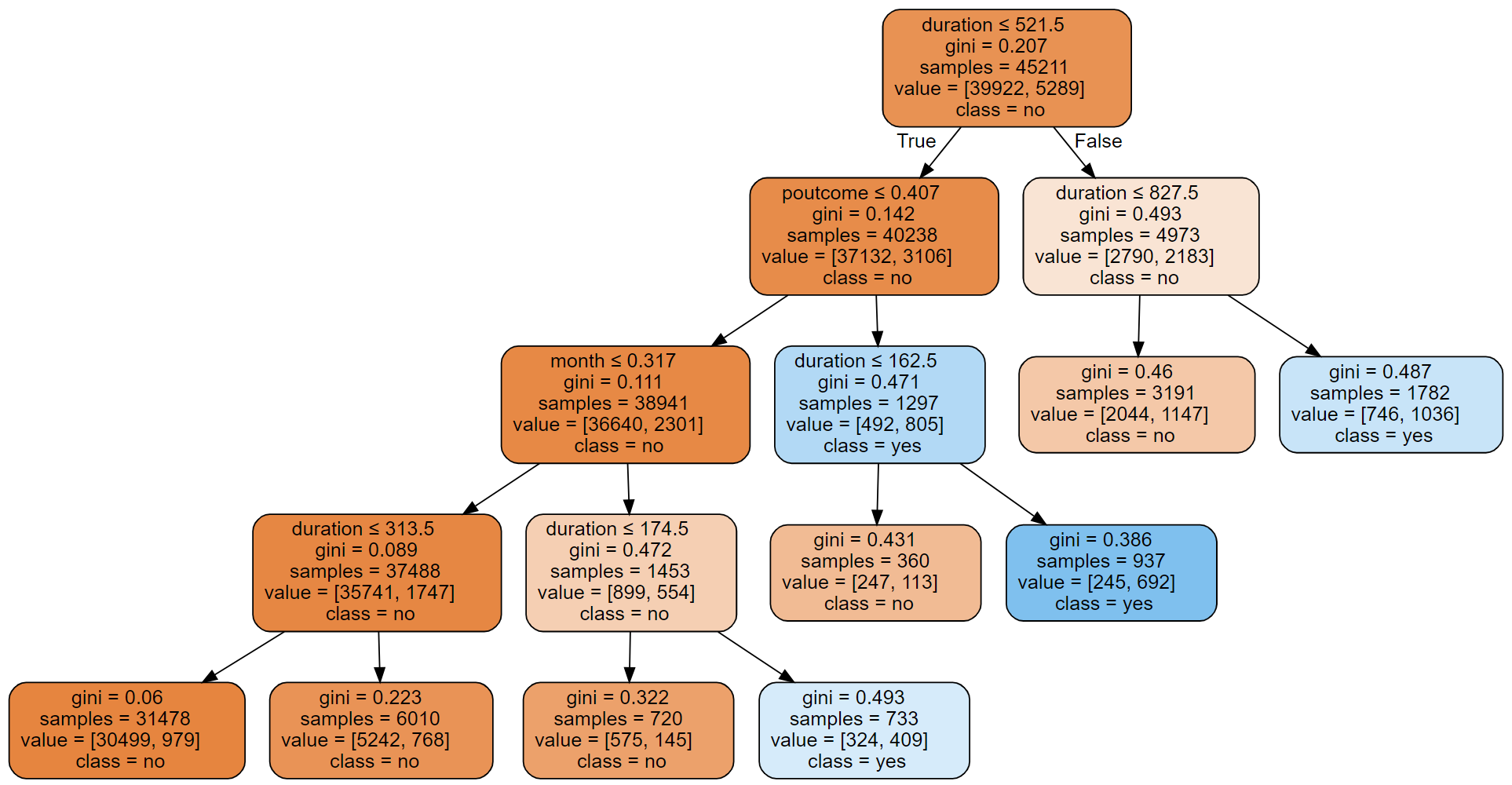
Exploration of independence between features: bivariate analysis
The variables that has the most influence on the target variable: duration(numerical feature), poutcome(categorical feature)
chi2 | mi | |||||||
---|---|---|---|---|---|---|---|---|
statistic | p-value | dof | dependence | mi | adjusted_mi | normalized_mi | ||
job | marital | 3837.6027 | 0.0000 | 22 | True | 0.0437 | 0.0287 | 0.0289 |
education | 28483.1365 | 0.0000 | 33 | True | 0.3069 | 0.1894 | 0.1896 | |
default | 60.3425 | 0.0000 | 11 | True | 0.0007 | 0.0005 | 0.0006 | |
housing | 3588.7309 | 0.0000 | 11 | True | 0.0411 | 0.0292 | 0.0293 | |
loan | 512.8105 | 0.0000 | 11 | True | 0.0069 | 0.0053 | 0.0054 | |
contact | 2047.1332 | 0.0000 | 22 | True | 0.0207 | 0.0139 | 0.0141 | |
month | 6043.8664 | 0.0000 | 121 | True | 0.0630 | 0.0297 | 0.0304 | |
poutcome | 559.2778 | 0.0000 | 33 | True | 0.0058 | 0.0039 | 0.0042 | |
y | 836.1055 | 0.0000 | 11 | True | 0.0083 | 0.0066 | 0.0067 | |
marital | education | 1337.5099 | 0.0000 | 6 | True | 0.0161 | 0.0158 | 0.0159 |
default | 16.7194 | 0.0002 | 2 | True | 0.0002 | 0.0003 | 0.0003 | |
housing | 19.3448 | 0.0001 | 2 | True | 0.0002 | 0.0002 | 0.0003 | |
loan | 121.9525 | 0.0000 | 2 | True | 0.0014 | 0.0020 | 0.0021 | |
contact | 183.8431 | 0.0000 | 4 | True | 0.0021 | 0.0023 | 0.0024 | |
month | 472.8791 | 0.0000 | 22 | True | 0.0052 | 0.0034 | 0.0035 | |
poutcome | 76.4791 | 0.0000 | 6 | True | 0.0008 | 0.0010 | 0.0011 | |
y | 196.4959 | 0.0000 | 2 | True | 0.0021 | 0.0033 | 0.0033 | |
education | default | 11.4246 | 0.0096 | 3 | True | 0.0001 | 0.0002 | 0.0002 |
housing | 643.8888 | 0.0000 | 3 | True | 0.0071 | 0.0078 | 0.0079 | |
loan | 291.3714 | 0.0000 | 3 | True | 0.0035 | 0.0044 | 0.0044 | |
contact | 1363.4366 | 0.0000 | 6 | True | 0.0151 | 0.0155 | 0.0156 | |
month | 1644.2895 | 0.0000 | 33 | True | 0.0178 | 0.0110 | 0.0113 | |
poutcome | 172.3951 | 0.0000 | 9 | True | 0.0019 | 0.0020 | 0.0022 | |
y | 238.9235 | 0.0000 | 3 | True | 0.0026 | 0.0035 | 0.0035 | |
default | housing | 1.5514 | 0.2129 | 1 | False | 0.0000 | 0.0000 | 0.0000 |
loan | 268.1092 | 0.0000 | 1 | True | 0.0024 | 0.0089 | 0.0089 | |
contact | 26.9295 | 0.0000 | 2 | True | 0.0003 | 0.0007 | 0.0007 | |
month | 155.6489 | 0.0000 | 11 | True | 0.0021 | 0.0019 | 0.0020 | |
poutcome | 73.8033 | 0.0000 | 3 | True | 0.0011 | 0.0029 | 0.0030 | |
y | 22.2022 | 0.0000 | 1 | True | 0.0003 | 0.0013 | 0.0013 | |
housing | loan | 76.9748 | 0.0000 | 1 | True | 0.0009 | 0.0015 | 0.0015 |
contact | 2062.4619 | 0.0000 | 2 | True | 0.0235 | 0.0312 | 0.0312 | |
month | 11494.0192 | 0.0000 | 11 | True | 0.1396 | 0.1024 | 0.1025 | |
poutcome | 926.4237 | 0.0000 | 3 | True | 0.0105 | 0.0156 | 0.0157 | |
y | 874.8224 | 0.0000 | 1 | True | 0.0097 | 0.0184 | 0.0184 | |
loan | contact | 11.9735 | 0.0025 | 2 | True | 0.0001 | 0.0002 | 0.0002 |
month | 1511.2025 | 0.0000 | 11 | True | 0.0155 | 0.0124 | 0.0125 | |
poutcome | 137.9993 | 0.0000 | 3 | True | 0.0019 | 0.0035 | 0.0035 | |
y | 209.6170 | 0.0000 | 1 | True | 0.0026 | 0.0065 | 0.0066 | |
contact | month | 23715.3268 | 0.0000 | 22 | True | 0.2971 | 0.2082 | 0.2083 |
poutcome | 3892.1528 | 0.0000 | 6 | True | 0.0625 | 0.0851 | 0.0852 | |
y | 1035.7142 | 0.0000 | 2 | True | 0.0136 | 0.0231 | 0.0232 | |
month | poutcome | 6230.9857 | 0.0000 | 33 | True | 0.0638 | 0.0472 | 0.0475 |
y | 3061.8389 | 0.0000 | 11 | True | 0.0244 | 0.0202 | 0.0203 | |
poutcome | y | 4391.5066 | 0.0000 | 3 | True | 0.0294 | 0.0581 | 0.0582 |
correlation | regression | ||||||||||
---|---|---|---|---|---|---|---|---|---|---|---|
pearson | p-pval | spearmanr | s-pval | kendalltau | k-pval | quasi-dependence | f | f-pval | quasi-dependence | ||
age | balance | 0.097783 | 1.846987e-96 | 0.096380 | 9.361066e-94 | 0.065226 | 6.014181e-93 | True | 436.437210 | 1.846987e-96 | True |
day | -0.009120 | 5.248053e-02 | -0.008948 | 5.709532e-02 | -0.006681 | 3.907395e-02 | True | 3.760582 | 5.248053e-02 | False | |
duration | -0.004648 | 3.229726e-01 | -0.033257 | 1.514473e-12 | -0.022444 | 1.784082e-12 | True | 0.976892 | 3.229726e-01 | False | |
campaign | 0.004760 | 3.114630e-01 | 0.037136 | 2.816751e-15 | 0.027816 | 2.757255e-15 | True | 1.024485 | 3.114630e-01 | False | |
pdays | -0.023758 | 4.367248e-07 | -0.017468 | 2.036697e-04 | -0.013679 | 2.356496e-04 | True | 25.532326 | 4.367248e-07 | True | |
previous | 0.001288 | 7.841413e-01 | -0.011900 | 1.139584e-02 | -0.009518 | 1.129966e-02 | True | 0.075037 | 7.841413e-01 | False | |
balance | day | 0.004503 | 3.383868e-01 | 0.001329 | 7.775064e-01 | 0.001242 | 6.982198e-01 | False | 0.916553 | 3.383868e-01 | False |
duration | 0.021560 | 4.545003e-06 | 0.042651 | 1.161677e-19 | 0.028586 | 1.086553e-19 | True | 21.025178 | 4.545003e-06 | True | |
campaign | -0.014578 | 1.936247e-03 | -0.030959 | 4.573514e-11 | -0.022924 | 4.563415e-11 | True | 9.610140 | 1.936247e-03 | True | |
pdays | 0.003435 | 4.651272e-01 | 0.069676 | 9.007228e-50 | 0.054180 | 4.248024e-49 | True | 0.533537 | 4.651272e-01 | False | |
previous | 0.016674 | 3.919530e-04 | 0.079536 | 2.361550e-64 | 0.062863 | 3.301276e-64 | True | 12.572057 | 3.919530e-04 | True | |
day | duration | -0.030206 | 1.327167e-10 | -0.058142 | 3.673297e-35 | -0.039337 | 8.186136e-35 | True | 41.287405 | 1.327167e-10 | True |
campaign | 0.162490 | 4.793707e-265 | 0.139581 | 1.892587e-195 | 0.105353 | 3.056587e-195 | True | 1226.027295 | 4.793707e-265 | True | |
pdays | -0.093044 | 1.764882e-87 | -0.092226 | 5.644265e-86 | -0.072813 | 1.148434e-84 | True | 394.801213 | 1.764882e-87 | True | |
previous | -0.051710 | 3.729346e-28 | -0.087780 | 4.944255e-78 | -0.070418 | 8.956173e-78 | True | 121.211875 | 3.729346e-28 | True | |
duration | campaign | -0.084570 | 1.521417e-72 | -0.107962 | 2.779222e-117 | -0.079976 | 3.223840e-117 | True | 325.663953 | 1.521417e-72 | True |
pdays | -0.001565 | 7.393560e-01 | 0.028698 | 1.040577e-09 | 0.022478 | 9.221545e-10 | True | 0.110695 | 7.393560e-01 | False | |
previous | 0.001203 | 7.981072e-01 | 0.031175 | 3.355401e-11 | 0.024689 | 2.785197e-11 | True | 0.065433 | 7.981072e-01 | False | |
campaign | pdays | -0.088628 | 1.621197e-79 | -0.112284 | 9.254439e-127 | -0.096802 | 1.246977e-125 | True | 357.921953 | 1.621197e-79 | True |
previous | -0.032855 | 2.794818e-12 | -0.108448 | 2.496456e-118 | -0.094371 | 3.617957e-117 | True | 48.854499 | 2.794818e-12 | True | |
pdays | previous | 0.454820 | 0.000000e+00 | 0.985645 | 0.000000e+00 | 0.902709 | 0.000000e+00 | True | 11791.089955 | 0.000000e+00 | True |
variance | correlation | ||||||||
---|---|---|---|---|---|---|---|---|---|
f | f-pval | quasi_dependence | spearmanr | s-pval | kendalltau | k-pval | quasi_dependence | ||
categorical | numerical | ||||||||
job | age | 1377.936493 | 0.000000e+00 | True | -0.008217 | 8.062405e-02 | -0.003349 | 3.241846e-01 | False |
balance | 43.007783 | 5.709430e-94 | True | 0.029609 | 3.036066e-10 | 0.021057 | 3.651549e-10 | True | |
day | 9.335477 | 5.489892e-17 | True | 0.022320 | 2.070497e-06 | 0.016542 | 1.230417e-06 | True | |
duration | 6.842766 | 1.232447e-11 | True | 0.005277 | 2.618159e-01 | 0.003780 | 2.596166e-01 | False | |
campaign | 12.483647 | 6.253473e-24 | True | 0.012609 | 7.337685e-03 | 0.009946 | 7.306402e-03 | True | |
pdays | 14.161079 | 1.107741e-27 | True | -0.008851 | 5.984944e-02 | -0.007197 | 6.621746e-02 | False | |
previous | 7.591359 | 3.183471e-13 | True | -0.002165 | 6.452470e-01 | -0.001853 | 6.396465e-01 | False | |
marital | age | 5228.732920 | 0.000000e+00 | True | -0.442815 | 0.000000e+00 | -0.354618 | 0.000000e+00 | True |
balance | 17.954318 | 1.605587e-08 | True | 0.020281 | 1.612420e-05 | 0.015796 | 2.068852e-05 | True | |
day | 1.348193 | 2.597196e-01 | False | -0.006203 | 1.871711e-01 | -0.004938 | 1.898360e-01 | False | |
duration | 12.078630 | 5.697950e-06 | True | 0.017361 | 2.229138e-04 | 0.013683 | 2.198743e-04 | True | |
campaign | 22.336983 | 2.013545e-10 | True | -0.030345 | 1.092802e-10 | -0.026402 | 1.140811e-10 | True | |
pdays | 19.695866 | 2.817855e-09 | True | 0.025644 | 4.942493e-08 | 0.023614 | 4.831779e-08 | True | |
previous | 6.550023 | 1.431440e-03 | True | 0.025697 | 4.637298e-08 | 0.023874 | 4.695176e-08 | True | |
education | age | 731.757745 | 0.000000e+00 | True | -0.115575 | 3.122264e-134 | -0.090377 | 1.833152e-133 | True |
balance | 116.682074 | 2.849538e-75 | True | 0.075328 | 6.801877e-58 | 0.058231 | 9.724104e-58 | True | |
day | 10.166018 | 1.089429e-06 | True | 0.024587 | 1.708703e-07 | 0.019347 | 1.587951e-07 | True | |
duration | 0.218271 | 8.837767e-01 | False | -0.003701 | 4.312879e-01 | -0.002875 | 4.281401e-01 | False | |
campaign | 6.617783 | 1.824042e-04 | True | -0.001645 | 7.265724e-01 | -0.001410 | 7.253331e-01 | False | |
pdays | 8.746901 | 8.522341e-06 | True | 0.026293 | 2.252637e-08 | 0.023545 | 2.804749e-08 | True | |
previous | 10.362132 | 8.192732e-07 | True | 0.034730 | 1.505560e-13 | 0.031632 | 1.510394e-13 | True | |
default | age | 14.456560 | 1.436177e-04 | True | -0.014681 | 1.798204e-03 | -0.012157 | 1.798915e-03 | True |
balance | 202.302934 | 8.246278e-46 | True | -0.167739 | 1.495206e-282 | -0.137371 | 1.345449e-278 | True | |
day | 4.015362 | 4.509349e-02 | True | 0.009727 | 3.862282e-02 | 0.008087 | 3.862420e-02 | True | |
duration | 4.540782 | 3.310185e-02 | True | -0.007100 | 1.311333e-01 | -0.005803 | 1.311318e-01 | False | |
campaign | 12.796137 | 3.476985e-04 | True | 0.014265 | 2.419778e-03 | 0.012894 | 2.420612e-03 | True | |
pdays | 40.668687 | 1.820913e-10 | True | -0.038053 | 5.780955e-16 | -0.036344 | 5.915284e-16 | True | |
previous | 15.193840 | 9.715925e-05 | True | -0.039279 | 6.554908e-17 | -0.037892 | 6.728462e-17 | True | |
housing | age | 1611.326374 | 0.000000e+00 | True | -0.154340 | 5.071808e-239 | -0.127809 | 3.390580e-236 | True |
balance | 214.812902 | 1.582632e-48 | True | -0.068292 | 7.020174e-48 | -0.055928 | 8.962332e-48 | True | |
day | 35.425150 | 2.669905e-09 | True | -0.027605 | 4.340367e-09 | -0.022951 | 4.367189e-09 | True | |
duration | 1.164622 | 2.805147e-01 | False | 0.005187 | 2.700684e-01 | 0.004240 | 2.700637e-01 | False | |
campaign | 25.190874 | 5.212410e-07 | True | -0.037807 | 8.877289e-16 | -0.034174 | 9.078130e-16 | True | |
pdays | 708.053596 | 8.305619e-155 | True | 0.080977 | 1.201289e-66 | 0.077341 | 1.950718e-66 | True | |
previous | 62.231686 | 3.121519e-15 | True | 0.062087 | 7.288802e-40 | 0.059896 | 8.608649e-40 | True | |
loan | age | 11.082880 | 8.719799e-04 | True | -0.004720 | 3.155434e-01 | -0.003909 | 3.155381e-01 | False |
balance | 323.965408 | 3.544641e-72 | True | -0.128966 | 6.474347e-167 | -0.105618 | 1.515890e-165 | True | |
day | 5.845397 | 1.562178e-02 | True | 0.012205 | 9.455904e-03 | 0.010147 | 9.457379e-03 | True | |
duration | 6.965838 | 8.310901e-03 | True | -0.013211 | 4.967530e-03 | -0.010798 | 4.968703e-03 | True | |
campaign | 4.503144 | 3.383802e-02 | True | 0.001587 | 7.357456e-01 | 0.001435 | 7.357415e-01 | False | |
pdays | 23.418093 | 1.307759e-06 | True | -0.029571 | 3.197051e-10 | -0.028243 | 3.223325e-10 | True | |
previous | 5.514300 | 1.886590e-02 | True | -0.030700 | 6.614993e-11 | -0.029617 | 6.678465e-11 | True | |
contact | age | 677.227898 | 1.597830e-290 | True | 0.053128 | 1.249988e-29 | 0.042091 | 1.565797e-28 | True |
balance | 55.110597 | 1.244231e-24 | True | -0.034245 | 3.256355e-13 | -0.027321 | 3.537308e-13 | True | |
day | 33.846140 | 2.050227e-15 | True | -0.027426 | 5.457756e-09 | -0.022262 | 5.305953e-09 | True | |
duration | 19.925809 | 2.239459e-09 | True | -0.036802 | 4.969902e-15 | -0.029422 | 4.272230e-15 | True | |
campaign | 70.326448 | 3.199084e-31 | True | 0.007996 | 8.909032e-02 | 0.007118 | 8.603679e-02 | False | |
pdays | 1486.235447 | 0.000000e+00 | True | -0.279500 | 0.000000e+00 | -0.260481 | 0.000000e+00 | True | |
previous | 550.425330 | 6.567538e-237 | True | -0.278906 | 0.000000e+00 | -0.262108 | 0.000000e+00 | True | |
month | age | 108.256036 | 3.059617e-245 | True | -0.032608 | 4.062113e-12 | -0.024028 | 2.017940e-12 | True |
balance | 102.277424 | 2.002290e-231 | True | 0.027575 | 4.512501e-09 | 0.018797 | 2.645153e-08 | True | |
day | 1052.969050 | 0.000000e+00 | True | 0.006697 | 1.544308e-01 | 0.009692 | 4.715761e-03 | True | |
duration | 19.028489 | 1.079679e-38 | True | 0.009111 | 5.270302e-02 | 0.006402 | 5.763659e-02 | False | |
campaign | 232.959857 | 0.000000e+00 | True | -0.147398 | 5.864119e-218 | -0.116475 | 3.733046e-214 | True | |
pdays | 365.011077 | 0.000000e+00 | True | 0.053558 | 4.388272e-30 | 0.043274 | 4.639729e-28 | True | |
previous | 130.342323 | 3.718515e-296 | True | 0.056224 | 5.486129e-33 | 0.047424 | 9.785824e-33 | True | |
poutcome | age | 26.381925 | 4.840702e-17 | True | 0.013266 | 4.790407e-03 | 0.010502 | 5.682389e-03 | True |
balance | 23.570292 | 3.088104e-15 | True | -0.075375 | 5.783112e-58 | -0.060154 | 9.550789e-58 | True | |
day | 113.814955 | 2.009226e-73 | True | 0.088062 | 1.591110e-78 | 0.071072 | 1.448078e-77 | True | |
duration | 31.136681 | 4.250760e-20 | True | -0.025125 | 9.140220e-08 | -0.019909 | 1.085663e-07 | True | |
campaign | 192.829765 | 2.888451e-124 | True | 0.116698 | 7.855655e-137 | 0.102844 | 6.674510e-136 | True | |
pdays | 51189.981633 | 0.000000e+00 | True | -0.990409 | 0.000000e+00 | -0.933486 | 0.000000e+00 | True | |
previous | 6179.512197 | 0.000000e+00 | True | -0.987244 | 0.000000e+00 | -0.925074 | 0.000000e+00 | True | |
y | age | 28.625233 | 8.825644e-08 | True | -0.008750 | 6.281716e-02 | -0.007246 | 6.281783e-02 | False |
balance | 126.572276 | 2.521114e-29 | True | 0.100295 | 2.095556e-101 | 0.082138 | 6.593767e-101 | True | |
day | 36.359010 | 1.653880e-09 | True | -0.029548 | 3.299041e-10 | -0.024566 | 3.326067e-10 | True | |
duration | 8333.761148 | 0.000000e+00 | True | 0.342469 | 0.000000e+00 | 0.279923 | 0.000000e+00 | True | |
campaign | 243.358404 | 1.012347e-54 | True | -0.084054 | 1.109367e-71 | -0.075977 | 1.948470e-71 | True | |
pdays | 490.696563 | 3.790553e-108 | True | 0.154055 | 3.900096e-238 | 0.147137 | 2.484050e-235 | True | |
previous | 396.443989 | 7.801830e-88 | True | 0.169124 | 2.852229e-287 | 0.163155 | 3.491720e-283 | True |
Descriptive statistics
- Class imbalanced categorical features: default, loan ,y
count | ratio | rank | self-information | |||||||
---|---|---|---|---|---|---|---|---|---|---|
total_count | column | unique | top | freq | entropy | instance | ||||
45211 | job | 12 | blue-collar | 9732 | 3.055353 | blue-collar | 9732 | 0.215257 | 1.0 | 2.215866 |
management | 9458 | 0.209197 | 2.0 | 2.257067 | ||||||
technician | 7597 | 0.168034 | 3.0 | 2.573172 | ||||||
admin. | 5171 | 0.114375 | 4.0 | 3.128159 | ||||||
services | 4154 | 0.091880 | 5.0 | 3.444101 | ||||||
retired | 2264 | 0.050076 | 6.0 | 4.319728 | ||||||
self-employed | 1579 | 0.034925 | 7.0 | 4.839591 | ||||||
entrepreneur | 1487 | 0.032890 | 8.0 | 4.926197 | ||||||
unemployed | 1303 | 0.028820 | 9.0 | 5.116765 | ||||||
housemaid | 1240 | 0.027427 | 10.0 | 5.188262 | ||||||
student | 938 | 0.020747 | 11.0 | 5.590942 | ||||||
unknown | 288 | 0.006370 | 12.0 | 7.294461 | ||||||
marital | 3 | married | 27214 | 1.315270 | married | 27214 | 0.601933 | 1.0 | 0.732325 | |
single | 12790 | 0.282896 | 2.0 | 1.821658 | ||||||
divorced | 5207 | 0.115171 | 3.0 | 3.118150 | ||||||
education | 4 | secondary | 23202 | 1.614902 | secondary | 23202 | 0.513194 | 1.0 | 0.962425 | |
tertiary | 13301 | 0.294198 | 2.0 | 1.765139 | ||||||
primary | 6851 | 0.151534 | 3.0 | 2.722287 | ||||||
unknown | 1857 | 0.041074 | 4.0 | 4.605628 | ||||||
default | 2 | no | 44396 | 0.130212 | no | 44396 | 0.981973 | 1.0 | 0.026244 | |
yes | 815 | 0.018027 | 2.0 | 5.793730 | ||||||
housing | 2 | yes | 25130 | 0.990985 | yes | 25130 | 0.555838 | 1.0 | 0.847263 | |
no | 20081 | 0.444162 | 2.0 | 1.170843 | ||||||
loan | 2 | no | 37967 | 0.634851 | no | 37967 | 0.839774 | 1.0 | 0.251928 | |
yes | 7244 | 0.160226 | 2.0 | 2.641815 | ||||||
contact | 3 | cellular | 29285 | 1.177525 | cellular | 29285 | 0.647741 | 1.0 | 0.626512 | |
unknown | 13020 | 0.287983 | 2.0 | 1.795944 | ||||||
telephone | 2906 | 0.064276 | 3.0 | 3.959567 | ||||||
month | 12 | may | 13766 | 2.937381 | may | 13766 | 0.304483 | 1.0 | 1.715564 | |
jul | 6895 | 0.152507 | 2.0 | 2.713051 | ||||||
aug | 6247 | 0.138174 | 3.0 | 2.855438 | ||||||
jun | 5341 | 0.118135 | 4.0 | 3.081492 | ||||||
nov | 3970 | 0.087810 | 5.0 | 3.509463 | ||||||
apr | 2932 | 0.064851 | 6.0 | 3.946717 | ||||||
feb | 2649 | 0.058592 | 7.0 | 4.093154 | ||||||
jan | 1403 | 0.031032 | 8.0 | 5.010087 | ||||||
oct | 738 | 0.016323 | 9.0 | 5.936909 | ||||||
sep | 579 | 0.012807 | 10.0 | 6.286967 | ||||||
mar | 477 | 0.010551 | 11.0 | 6.566541 | ||||||
dec | 214 | 0.004733 | 12.0 | 7.722919 | ||||||
poutcome | 4 | unknown | 36959 | 0.937015 | unknown | 36959 | 0.817478 | 1.0 | 0.290748 | |
failure | 4901 | 0.108403 | 2.0 | 3.205526 | ||||||
other | 1840 | 0.040698 | 3.0 | 4.618896 | ||||||
success | 1511 | 0.033421 | 4.0 | 4.903098 | ||||||
y | 2 | no | 39922 | 0.520631 | no | 39922 | 0.883015 | 1.0 | 0.179490 | |
yes | 5289 | 0.116985 | 2.0 | 3.095607 |
column | count | norm_statstic | norm_pval | normality | l_shift | r_shift | iqr_min | iqr_25 | mean | iqr_75 | iqr_max | std | diff_maxmin | |
---|---|---|---|---|---|---|---|---|---|---|---|---|---|---|
0 | age | 45211.0 | 3066.989468 | 0.0 | False | True | False | 18.0 | 33.0 | 40.936210 | 48.0 | 95.0 | 10.618762 | 77.0 |
1 | balance | 45211.0 | 64697.210210 | 0.0 | False | True | False | -8019.0 | 72.0 | 1362.272058 | 1428.0 | 102127.0 | 3044.765829 | 110146.0 |
2 | day | 45211.0 | 14624.380064 | 0.0 | False | False | True | 1.0 | 8.0 | 15.806419 | 21.0 | 31.0 | 8.322476 | 30.0 |
3 | campaign | 45211.0 | 45156.283654 | 0.0 | False | True | False | 1.0 | 1.0 | 2.763841 | 3.0 | 63.0 | 3.098021 | 62.0 |
4 | pdays | 45211.0 | 24050.969837 | 0.0 | False | True | False | -1.0 | -1.0 | 40.197828 | -1.0 | 871.0 | 100.128746 | 872.0 |
5 | previous | 45211.0 | 134066.595245 | 0.0 | False | True | False | 0.0 | 0.0 | 0.580323 | 0.0 | 275.0 | 2.303441 | 275.0 |
Description for attributes
- age
- job : type of job
- marital : marital status
- education
- default: has credit in default?
- housing: has housing loan?
- loan: has personal loan?
- contact: contact communication type
- month: last contact month of year
- day_of_week: last contact day of the week
- duration: last contact duration, in seconds (numeric). Important note: this attribute highly affects the output target (e.g., if duration=0 then y='no'). Yet, the duration is not known before a call is performed. Also, after the end of the call y is obviously known. Thus, this input should only be included for benchmark purposes and should be discarded if the intention is to have a realistic predictive model.
- campaign: number of contacts performed during this campaign and for this client (numeric, includes last contact)
- pdays: number of days that passed by after the client was last contacted from a previous campaign (numeric; 999 means client was not previously contacted)
- previous: number of contacts performed before this campaign and for this client (numeric)
- poutcome: outcome of the previous marketing campaign (categorical: 'failure','nonexistent','success') # social and economic context attributes
- y - has the client subscribed a term deposit? (binary target: 'yes','no')
Data Extraction
age | job | marital | education | default | balance | housing | loan | contact | day | month | duration | campaign | pdays | previous | poutcome | y | |
---|---|---|---|---|---|---|---|---|---|---|---|---|---|---|---|---|---|
0 | 58 | management | married | tertiary | no | 2143 | yes | no | unknown | 5 | may | 261 | 1 | -1 | 0 | unknown | no |
1 | 44 | technician | single | secondary | no | 29 | yes | no | unknown | 5 | may | 151 | 1 | -1 | 0 | unknown | no |
2 | 33 | entrepreneur | married | secondary | no | 2 | yes | yes | unknown | 5 | may | 76 | 1 | -1 | 0 | unknown | no |
3 | 47 | blue-collar | married | unknown | no | 1506 | yes | no | unknown | 5 | may | 92 | 1 | -1 | 0 | unknown | no |
4 | 33 | unknown | single | unknown | no | 1 | no | no | unknown | 5 | may | 198 | 1 | -1 | 0 | unknown | no |
5 | 35 | management | married | tertiary | no | 231 | yes | no | unknown | 5 | may | 139 | 1 | -1 | 0 | unknown | no |
6 | 28 | management | single | tertiary | no | 447 | yes | yes | unknown | 5 | may | 217 | 1 | -1 | 0 | unknown | no |
7 | 42 | entrepreneur | divorced | tertiary | yes | 2 | yes | no | unknown | 5 | may | 380 | 1 | -1 | 0 | unknown | no |
8 | 58 | retired | married | primary | no | 121 | yes | no | unknown | 5 | may | 50 | 1 | -1 | 0 | unknown | no |
9 | 43 | technician | single | secondary | no | 593 | yes | no | unknown | 5 | may | 55 | 1 | -1 | 0 | unknown | no |
Missing value & Duplication inspection
column | total | missing-value | duplication | |||||||
---|---|---|---|---|---|---|---|---|---|---|
quasi-dtypes | freq | not_freq | freq | ratio | rank | cardinality | selectivity | rank | ||
0 | age | numeric | 45211 | 45211 | 0 | 0.0 | 9.0 | 77 | 0.001703 | 14.0 |
1 | job | string | 45211 | 45211 | 0 | 0.0 | 9.0 | 12 | 0.000265 | 9.5 |
2 | marital | string | 45211 | 45211 | 0 | 0.0 | 9.0 | 3 | 0.000066 | 5.5 |
3 | education | string | 45211 | 45211 | 0 | 0.0 | 9.0 | 4 | 0.000088 | 7.5 |
4 | default | string | 45211 | 45211 | 0 | 0.0 | 9.0 | 2 | 0.000044 | 2.5 |
5 | balance | numeric | 45211 | 45211 | 0 | 0.0 | 9.0 | 7168 | 0.158545 | 17.0 |
6 | housing | string | 45211 | 45211 | 0 | 0.0 | 9.0 | 2 | 0.000044 | 2.5 |
7 | loan | string | 45211 | 45211 | 0 | 0.0 | 9.0 | 2 | 0.000044 | 2.5 |
8 | contact | string | 45211 | 45211 | 0 | 0.0 | 9.0 | 3 | 0.000066 | 5.5 |
9 | day | numeric | 45211 | 45211 | 0 | 0.0 | 9.0 | 31 | 0.000686 | 11.0 |
10 | month | string | 45211 | 45211 | 0 | 0.0 | 9.0 | 12 | 0.000265 | 9.5 |
11 | duration | numeric | 45211 | 45211 | 0 | 0.0 | 9.0 | 1573 | 0.034792 | 16.0 |
12 | campaign | numeric | 45211 | 45211 | 0 | 0.0 | 9.0 | 48 | 0.001062 | 13.0 |
13 | pdays | numeric | 45211 | 45211 | 0 | 0.0 | 9.0 | 559 | 0.012364 | 15.0 |
14 | previous | numeric | 45211 | 45211 | 0 | 0.0 | 9.0 | 41 | 0.000907 | 12.0 |
15 | poutcome | string | 45211 | 45211 | 0 | 0.0 | 9.0 | 4 | 0.000088 | 7.5 |
16 | y | string | 45211 | 45211 | 0 | 0.0 | 9.0 | 2 | 0.000044 | 2.5 |
References
'quantitative analysis > analysis report' 카테고리의 다른 글
[Regression] Air Quality (2) | 2023.05.07 |
---|